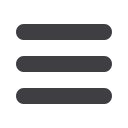
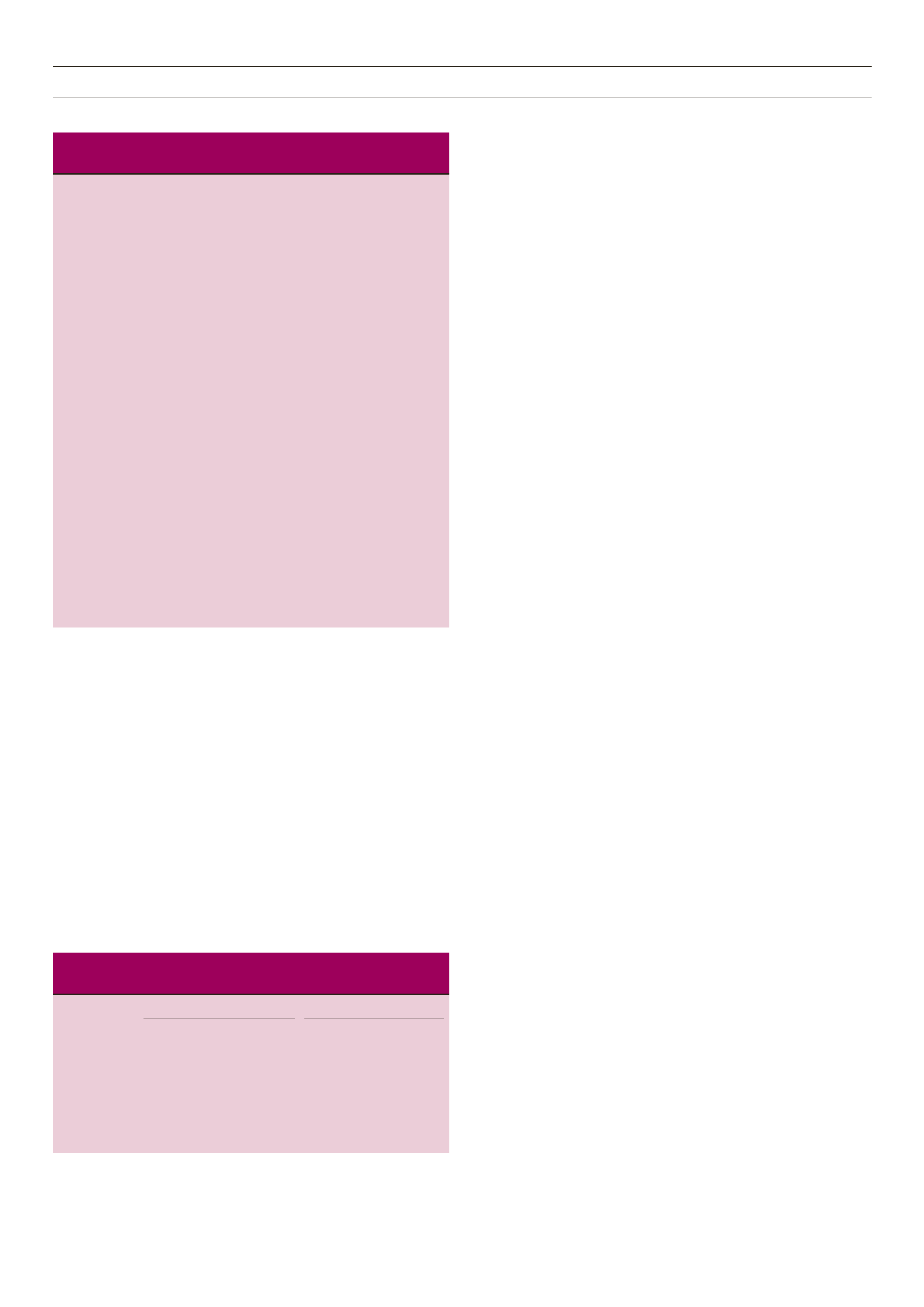
SA JOURNAL OF DIABETES & VASCULAR DISEASE
RESEARCH ARTICLE
VOLUME 15 NUMBER 2 • NOVEMBER 2018
49
Multivariate analysis was done using a forward stepwise binary
logistic regression in order to assess for independent predictors
of hypertension and abnormal blood glucose levels. We included
predictor variables with associations at a significance level of
p
≤
0.2 on univariate analysis in order to accommodate for important
risk factors. The final logistic regression model (Table 5) showed that
as age and BMI increased, the chances of becoming hypertensive
increased 1.09 and 2.99 times (OR 1.09; 95% CI: 1.06–1.1,
p
<
0.0001; OR 2.99; 95% CI: 1.69–5.31,
p
< 0.0001), respectively.
For abnormal glucose level with increasing BMI, the chances of
having abnormal glucose level increased 2.5 times (OR 2.39; 5%
CI: 1.33–4.30;
p
= 0.004). Other variables such as physical activity,
number of driving hours, waist circumference and professional
driving years were not independently associated with our outcome
parameters and were excluded from the final regression model.
Discussion
The major finding of this study was that male long-distance bus
drivers had a higher prevalence of clustering of cardiometabolic risk
factors than the general population, and in addition, most them
were unaware of their risk status.
12,14
This clustering places them at
a higher risk for CVD and contributes significantly to the already
burgeoning CVD burden in the general population. Importantly, a
CVD event in a driver while driving portends grave danger to him,
the passengers and other road users.
The prevalence of hypertension in this study was 39.7%, with
75.9%being newly diagnosed. This is higher than the recent pooled
national prevalence rate of 28.9% but lower than the 44.9%
prevalence from a national study on blindness and hypertension.
32,33
Previous local studies reported prevalence rates ranging from 21.4
to 33.5%.
19-21
Studies from Brazil and Iran reported prevalence rates
of 45.6 and 44.6%, respectively, much higher than their national
prevalence rates.
12,13
Professional drivers, by nature of their occupation, are largely
sedentary and indulge in dietary indiscretions, which could lead to
obesity. From this study, obesity was a predictor of hypertension.
Furthermore, BMI and longer duration of years of professional
driving significantly correlated with the risk of hypertension,
similar to findings by Sangaletti
et al
.
12
This association is plausible,
as drivers who drive for long hours over many years tend to gain
weight inappropriately due to physical inactivity and dietary
indiscretion.
In addition to high prevalence of hypertension, optimal blood
pressure control was equally low among the subjects. Among the
9.6% previously known hypertensives, only 21.4% had optimal
BP control. BP control is generally very low in Nigeria, ranging
between five and 29.4%.
34,35
Ignorance, long travel times, poor
access to standard medical care, the asymptomatic nature of
hypertension and the relative lack of self-care among males have
been suggested as possible causes of poor BP control among
long-distance drivers.
12
The prevalence of abnormal glucose profiles in this study was
45.2%, comprising 31.3 and 13.9% for impaired fasting gliucose
levels and diabetes mellitus (DM), respectively. Most of the
diabetics were diagnosed for the first time during this study. There
are no local studies for comparison but the reported prevalence of
DM from this study is much higher than the 4.5% reported by the
International Diabetes Federation (IDF) and the eight to 10% from
a study on the general population.
36,37
In Iran, the prevalence of
DM among drivers was 17.5%, comparable to the value obtained
from this study, but higher than the 8.5% prevalence reported by
the IDF in 2014.
13
Obesity is a risk factor for type 2 DM. From our
study, BMI was a predictor of abnormal glucose profiles, similar to
the findings by Sangaletti
et al
.
12
The prevalence of dyslipidaemia in this study was 56.3%,
comparable to the national average of 60.1%.38 The predominant
dyslipidaemia was elevated TC levels in 27.8% of the subjects,
followed by elevated LDL-C levels in 24.6%, elevated triglycerides
in 24.6% and low HDL-C levels in 6.5%. There are no local
studies of lipid abnormalities in professional drivers. The pattern
obtained is at variance however with patterns reported in local
studies in apparently healthy Nigerians, in which the predominant
dyslipidaemia was low HDL-C levels.
38
In Iran, professional drivers
have been shown to have predominantly hypertriglyceridaemia and
central obesity, attributable to stressful working conditions.
13
Table 4.
Association between independent variables and hypertension
and abnormal glucose levels
Hypertension
Abnormal glucose levels
Parameter
% (95% CI)
p
-value % (95% CI)
p
-value
Driving hours/week
0.250
0.076
≥ 36
42.9 (35.0–50.9)
35.6 (27.9–43.2)
< 36
36.3 (28.5–44.2)
25.9 (18.6–33.2)
Years of professional
driving
< 0.001
0.320
≥ 20
56.2 (43.1–64.4)
33.1 (25.4–40.8)
< 20
23.1 (16.2–30.0)
27.7 (20.3–35.0)
Physical activity
0.279
0.205
< 600 METs/week 42.6 (34.6–50.5)
27.6 (20.3-34.9)
≥ 600 METs/week 36.3 (28.5–44.2)
34.5 (26.7-42.3)
BMI
< 0.001
0.002
Overweight/obese 48.4 (41.1–55.6)
37.8 (30.7–44.9)
Normal
25.9 (17.7–34.2)
19.8 (12.2–27.4
Alcohol use
0.840
0.807
Yes
40.1 (33.4–46.8)
31.2 (24.9–37.6)
No
38.8 (28.5–49.2)
29.8 (20.0–39.5)
Smoking
0.477
0.808
Yes
43.9 (31.0–56.7)
32.1 (19.9-44.4)
No
38.7 (28.5-49.2)
30.5 (24.6-36.4)
WC (cm)
< 0.001
0.076
> 102
61.4 (50.0–72.8)
39.7 (28.1–51.3)
≤ 102
33.0 (26.8–39.2)
28.3 (22.3–34.3)
Age
< 0.001
0.499
≥ 45
54.5 (46.4–62.6)
32.6 (25.0–40.3)
< 45
25.2 (18.2–32.2)
29.0 (21.6–36.3)
BMI: body mass index; WC: waist circumference; METs: metabolic
equivalents.
Table 5.
Logistic regression on predictors of hypertension and abnormal
glucose levels
Hypertension
a
Abnormal glucose levels
b
Variables
OR (95% CI)
p
-value
OR (95% CI)
p
-value
Age
1.090 (1.058–1.23) < 0.0001
ns
ns
Overweight/
2.99 (1.69–5.32) < 0.0001 2.39 (1.33–4.3)
0.04
obesity
a
Variables excluded from the final model were: physical activity, number of
driving hours, waist circumference and professional driving years.
b
Variables excluded from the final model were: age, physical activity, number
of driving hours, waist circumference and professional driving years.