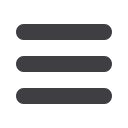
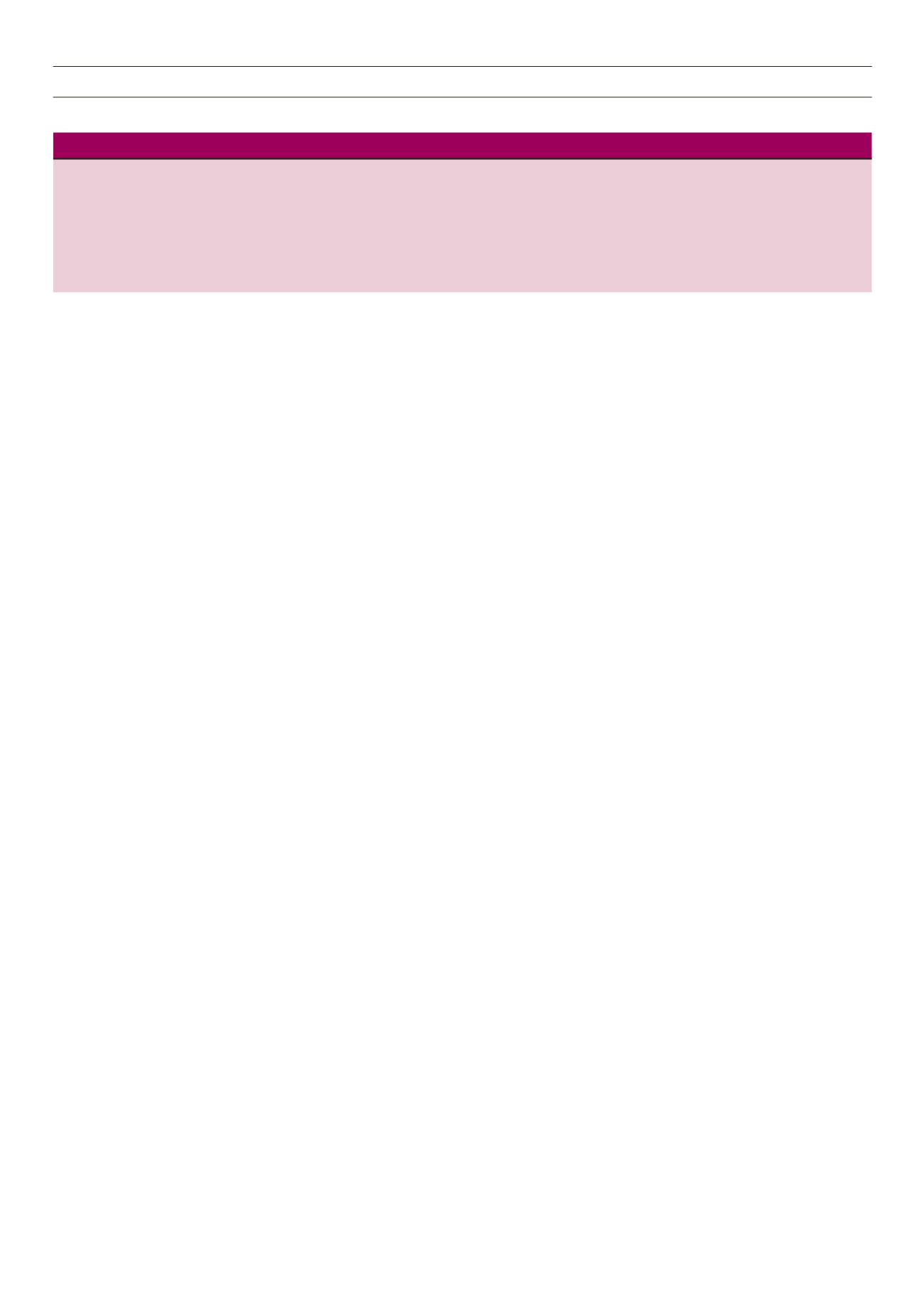
SA JOURNAL OF DIABETES & VASCULAR DISEASE
RESEARCH ARTICLE
VOLUME 17 NUMBER 1 • JULY 2020
7
determine correlation between GDM diagnosed by the WHO and
GDM diagnosed by IADPSG criteria, and it was found to be 0.597
(a coefficient of zero being no correlation and a coefficient of one
being a strong correlation).
Table 4 shows risk factors associated with GDM. Five per cent
of the women reported having previously had macrosomic babies
(birth weight > 4 kg). This could not be confirmed as the majority
did not have written records of birth weights from previous
pregnancies and their responses were based on recall. Seven
per cent reported having first-degree relatives with diabetes and
19% reported having had miscarriages or stillbirths in previous
pregnancies.
Attending government ANCs, age and parity were associated
with having GDM (
p
< 0.05). The risk of having GDM was higher in
government compared to private ANCs and this increased with age
and parity. A family history of diabetes mellitus, previous miscarriage/
stillbirth, BMI, being HIV positive and having hypertension were not
associated with GDM (
p
> 0.05).
Seventy-one per cent of the women diagnosed with GDM
were lost to follow up post-delivery and complete outcome data
were available for only 18 women. There were four miscarriages,
four women who had a caesarean section and two babies with
macrosomia. Data on follow up for diabetes at six weeks postpartum
in particular were missing as this was collected telephonically and
some of the women could not be reached.
Discussion
This study showed that the prevalence of gestational diabetes
mellitus in Blantyre was low. It also showed a wide discrepancy in
the prevalence when IADPSG criteria were used compared to WHO
criteria, with a 12-fold increase in the prevalence when the IADPSG
criteria were used. To our knowledge, this is the first description of
the prevalence of gestational diabetes in the Malawian population.
The HAPO study, with an average BMI of 27 kg/m
2
among its
participants, showed a direct correlation between obesity and poor
outcomes.
24
Our study population, however, being largely young
with few obese women (1% based on MUAC), was different from
that described in other studies of risk factors for GDM.
In the nationwide WHO STEPS survey,
13
the prevalence of
overweight and obesity among Malawian women was 16 and 2%,
respectively. The age of the women screened was 25–64 years, but
the majority of the women screened were young, as 46% of the
women were between the ages of 25 and 34 years. Our GDM study
similarly screened a young population of women and the prevalence
of overweight and obesity was 9 and 1%, respectively. From both
studies, obesity appears to be rare among Malawian women.
In another 2007 study of 620 patients attending the adult
diabetes clinic at QECH, the average BMI in type 2 DM patients
was 28.7 kg/m
2
.
25
These observations suggest that obesity may not
be the main driver for the DM epidemic in Malawi and that other
factors such as genetics, low birth weight and stunting may play a
larger role.
Advanced maternal age, high parity and attending government
ANCs were associated with GDM, the older women being more
likely to have high parity than the younger, consistent with
traditional risk factors for GDM. Other known risk factors for GDM,
such as a family history of DM, a history of macrosomia, previous
miscarriages or stillbirths, or MUAC were not associated with GDM.
As observed in the STEPS survey, the majority of DM in the
population is undiagnosed; as such a negative family history of
DM may in part be a reflection of this. The overall picture however
highlights the fact that risk factors for developing GDM may be
population specific and there may be genetic variability inherent in
the population to explain such differences. This raises a need for
exploring population-specific risk factors other than those stated in
the WHO guidelines or those from high-income countries.
Women attending private hospitals are generally perceived as
having a higher socio-economic status and more likely to adopt
a diet rich in refined foods and a sedentary lifestyle than their
counterparts. By including private ANCs, we anticipated showing
that this group would tend to be more obese and have a higher
risk of developing GDM. Our findings though were contrary to this
expectation as there was no difference in terms of nutritional status
between women from government facilities and those from private
hospitals. Furthermore, women at private ANCs were less likely to
have GDM than those in government hospitals.
Dietary differences between the two groups were not explored
in particular but it appears that the risk that may be conferred
by sedentary habits or a Westernised diet may be balanced by
better health-seeking behaviour and ready access to screening and
diagnostic services in private hospitals.
RBG measurements were largely normal as only three women
had RBG levels > 11.1 mmol/l and 75% of the study population
had an RBG level below 5.5 mmol/l. Other than the RBG test being
an insensitive screening tool, it was also observed on random
questioning that many of the women at the health centres had not
eaten for some time before the measurement, particularly those
who had to leave their homes early in the morning to attend the
clinic on time. Their results may reflect a fasting rather than RBG
level and may explain the large proportion of women with normal
RBG levels. There was no correlation between RBG level and GDM
diagnosed by OGTT or risk factors for GDM. The RBG test may
therefore not be a sensitive screening tool or used as a proxy for
OGTTs in this population.
The prevalence of GDM of 1.6% using WHO criteria was lower
than that described in other African studies using the 1999 WHO
Table 4.
Risk factors associated with GDM by WHO criteria
Parameter
df
Estimate
Standard error
Wald chi-square
p
-value
Government ANCs
1
2.0860
0.5959
12.2527
0.0005
Age
1
0.0973
0.0424
5.2690
0.0217
Parity
1
0.6160
0.2353
6.8541
0.0088
MUAC
1
–0.0744
0.0533
1.9534
0.1622
Previous macrosomia
1
–1.8416
0.9789
3.5391
0.059
df, degrees of freedom, ANC, antenatal clinic; GDM, gestational diabetes mellitus; MUAC, mid upper-arm circumference.